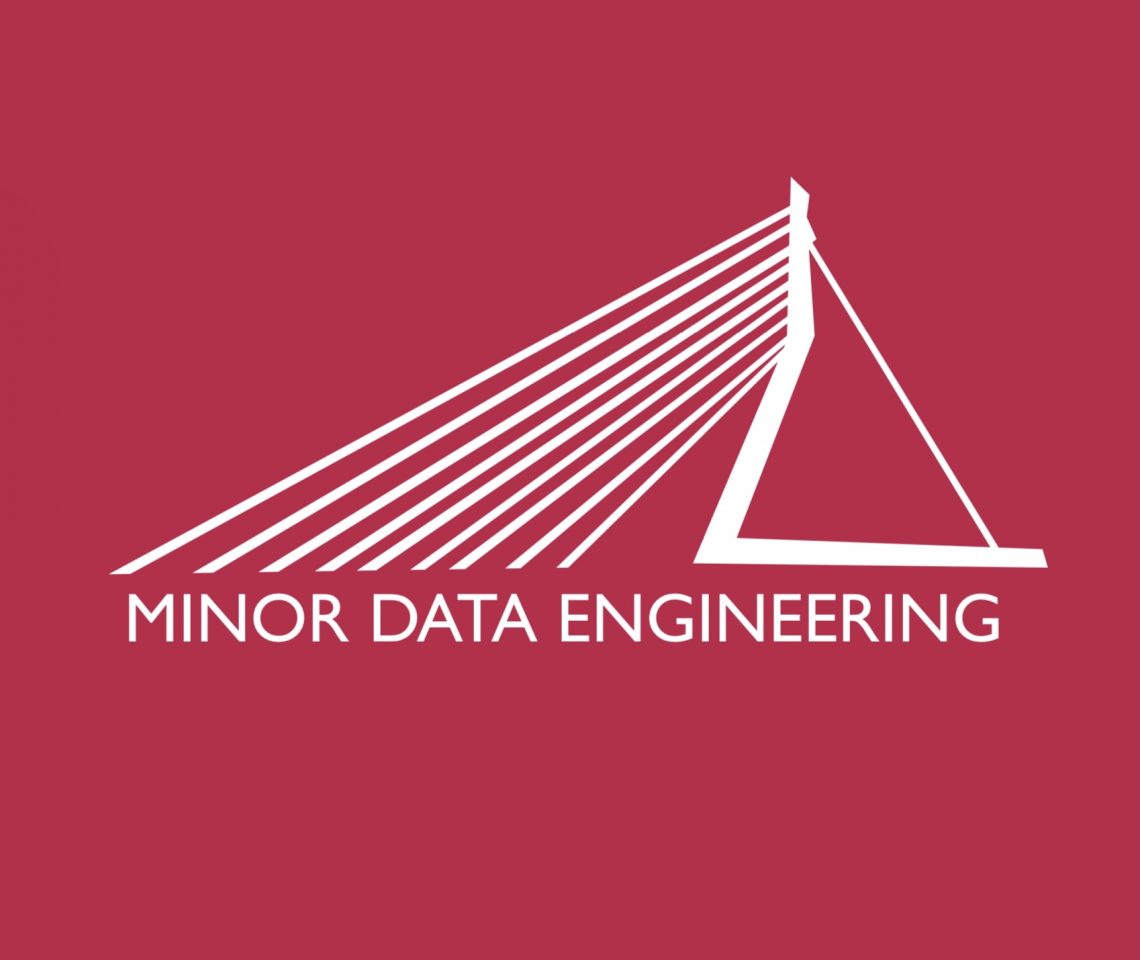
The birth of Data engineering
In 2017 I wanted to create a minor on Business Intelligence (BI). Unfortunately, the mind of my manager was already polluted into thinking BI was just about creating reports. And thus unfit to complement a software engineering program.
I disagreed, of course, but there was no persuasion on the horizon. So I decided to rebrand the BI I had envisioned. In my humble opinion BI is so much more than just reporting. In fact: the report is the most boring part of the art.
The real mastery is found in creating a solid, robust, auditable and flexible data environment, that can be used to create reports, but also for analysis, machine learning, data science, and whatever we think of next to do with reliable data.
It had to sound technical enough to convey the need for software engineering skills, but also emphasise the importance of software architecture knowledge, modelling knowledge (not just an ERD), just plain experience (at least an internship).
And on the more skill side of things: knowledge about legislation, on ethics, dealing with non-it customers.
I brainstormed on it with a colleague and we came up with: ’the engineering of data value chains’. It did cover it well enough, but it was a bit underwhelming.
They say you get the best ideas while sleeping. In this case they where right. The next morning I knew: Data Engineering. It sounded technical enough and the word data in front conveyed enough to separate it from software engineering.
I discussed it with my colleague and he agreed. Thus Data Engineering was born!
Because I hate to create education nobody is waiting for, I started talks with colleagues in companies I worked for or worked with to discuss my plan. Later I created a Meetup group (Rotterdam Data Engineering) to verify my plans and ideas. I discussed the progress on LinkedIn frequently and invited my network to attend and in February 2019 my dream was ready: the first edition of the Minor Data Engineering.
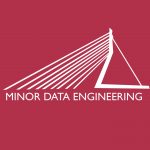
After starting the minor, students came to me, they found different definitions of data engineering on the web. To my astonishment, people had not only adopted the term, and found their own definition, but started to recognise it as a separate profession in the data field. You can imagine my glee at this discovery.
One of my sponsors at Informatica.com called it the most secret job in the field. Apparently I wasn’t the only one to try to distinguish between data science and what we now call data engineering. It really needs a very different skill set.
In my opinion data engineering can be loosely defined as:
” All things that need to be done to be able to (re)use data in a meaningful way.”
With this definition, you cover all that is needed for a solid, robust, audit-able and flexible data foundation. You will know your data through organised meta data, and therefore be able to use it.
In the minor we use the ‘quadrants’ model created by Ronald Damhof to express the actions needed.
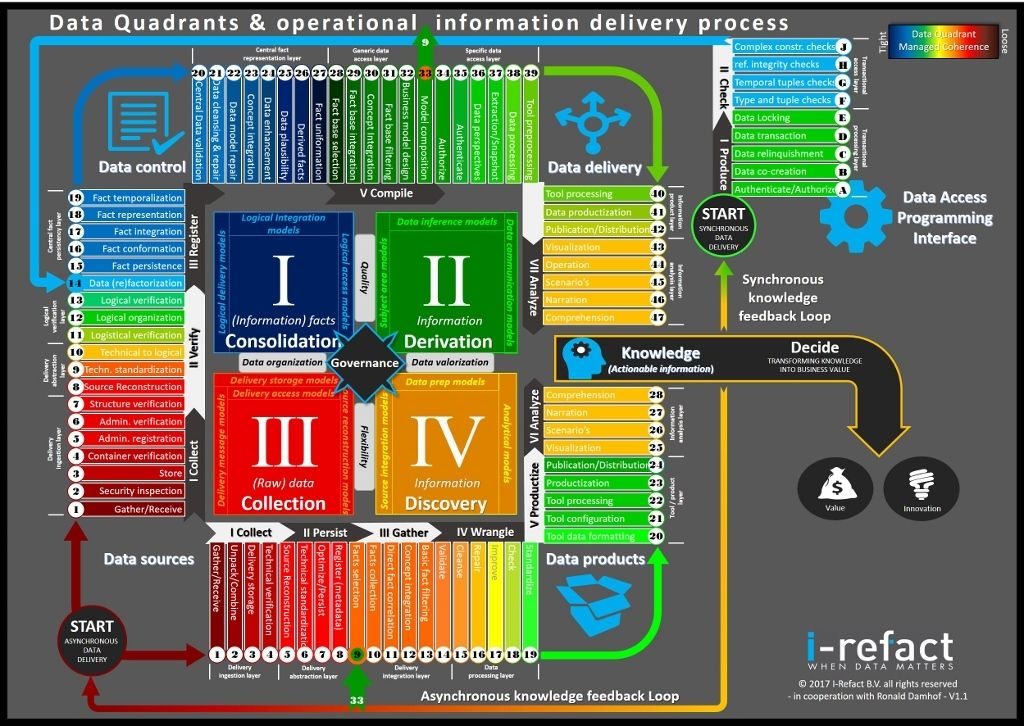

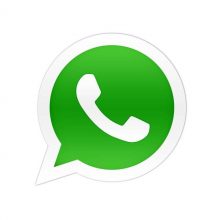
2 Comments
hans.konstapel
I startted to work in 1976 at ABN as an Operation Research Analist, My education at that time started at 1969 and was called Mathematics. One of the new parts of mathematics was called Language Theory (Chomsky etc) and we learned programming (in Algol) and Statistics and Numerical Analysis. What s called Data Engineering is far behind what we already knew and practiced 50 years ago. The only difference is the power of the processor which caused the end of thinking for yourself and a terrible waste of energy caused by computers. My advice is to go back in time and read the fundamentals so you are not degraded to a botton-pusher.
tanja
I know, I started working in 1987 and got in to IT in 1989, but it is about the birth of the call sign, not the field. Being in IT as long as I am, and from your note I think you are in IT a long time too, it is always new names for the same mathematical concepts. Just faster computers and bigger networks.
But as a lecturer, my students don’t have such an elaborate background and they think it is a funny story that we invented the term. That’s why I committed it to this blog. Going in to the integrate history of how computers came to be, is not a thing that is addressed regularly anymore.